Understanding Artificial Intelligence In Medicine: The Future Of Healthcare
The term “artificial intelligence” was first coined by John McCarthy in 1956 as the science and engineering of making human-level intelligent machines. Fast-forward 70 years, and artificial intelligence has become integral to our daily lives.
Artificial intelligence has transformed various industries, including entertainment, transportation, and healthcare. In medical settings, AI is used to help process medical data and assist healthcare professionals in diagnosis, improving patient outcomes and care quality.
This article aims to provide a comprehensive view of AI in medicine. We’ll explore artificial intelligence applications in modern medicine and speculate on their impacts on the future of patient care.
Artificial Intelligence Adoption in Today’s Healthcare
Artificial intelligence (AI) has become a major focus across various sectors. Thanks to advancements in computer science and informatics, new AI algorithms and AI-powered applications are introduced every day.

However, the adoption of AI in medicine and patient care is less robust. Artificial intelligence mainly plays a support role in today’s clinical settings, aiding medical professionals in decision-making and research.
Some of the popular AI-powered solutions in medicine include:
- Image Analysis and Interpretation: AI tools are trained to detect patterns and identify lesions or other irregularities in CT scans, x-rays, and MRIs. Deep learning models like convolutional neural networks (CNNs) allow AI algorithms to efficiently locate anomalies among complex details in medical images that might be challenging for human eyes to notice.
- Operational Efficiency Enhancement: AI significantly enhances image interpretation and data analysis efficiency and speed. Its capacity to accurately process large medical information eliminates the need for repeat scans and diagnoses. A study shows that when aided by AI, the average assessment time per case shrinks from 2:44 minutes to 35.7 seconds, reducing healthcare costs.
- Predictive Analytics: Artificial intelligence facilitates precision medicine. Aided by electronic health records (EHR), AI algorithms can access patients’ past health data to predict risks and support clinical decisions. For example, a breast cancer prediction algorithm was trained on 38,444 mammogram images from 9,611 women. It was able to predict biopsy malignancy at a level comparable to radiologists.
- Clinical Decision Support: AI aids medical professionals in the decision-making process by providing valuable insights. Clinical Decision Support Systems (CDSS) use AI technologies to process and interpret vast amounts of healthcare data to discern patterns, recognize correlations, and derive actionable insights.
Of course, implementing AI in medicine has drawn concerns, which we’ll address later. However, we cannot deny its benefits in contemporary healthcare. As more doctors, clinicians, and researchers adopt AI technologies, we may see AI integrate seamlessly into healthcare systems and evolve beyond the current supportive role.
Examples of AI Technologies in Medicine
The application of AI in medicine may not be as prevalent as in other parts of life. It often runs in the background, speeding up clinical decision-making and medical research. Here are two prime examples of how AI technologies are applied in medical and healthcare settings.
AI-based Predictive Model Helps Validate Benefits of Hormone Therapy for Prostate Cancer Patients
While androgen deprivation therapy (ADT) offers documented benefits for localized prostate cancer treatment, it may also have adverse impacts on quality of life. Scientists developed an AI-based predictive model to identify prostate cancer patients who would benefit from adding ADT to radiotherapy.

The study looked at digital pathology images and clinical data from 5,727 patients in five big trials that included radiotherapy, with or without ADT. The AI model was trained to predict which patients might develop distant metastasis, giving a yes or no answer on whether ADT would be helpful.
They tested the AI with data from another trial, RTOG 9408, where patients were randomly given radiotherapy with or without short-term ADT. The AI model found a group of patients, specifically 34%, who would benefit from short-term ADT by lowering their risk of distant metastasis, while others wouldn't see the same benefit.
This AI-based model provides a more reliable way to determine which prostate cancer patients could benefit from ADT, possibly saving many from its side effects. It’s a step up from the usual risk assessment methods using AI. However, more research is needed to see how it works in different groups and settings.
AI Assistance in Breast Cancer Diagnostics
A study in the UK used an AI system to interpret a large dataset of mammograms. The AI model produced diagnostics with an absolute reduction in false positives and false negatives by 5.7% and 9.4%, respectively.
The AI system outperformed six radiologists. It achieved a higher area under the receiver operating characteristic curve (AUC-ROC), surpassing the average radiologist's performance by 11.5%.
In another study in South Korea, an artificial intelligence diagnostic model analyzed 170,230 mammograms. The AI system detected 90% of breast cancers with mass, compared to 78% identified by radiologists. It was also notably better at identifying early-stage cancers.
AI's integration into breast cancer screening holds promise. Not only does it improve accuracy, but it also reduces the workload of second readers by 88%. This efficiency could pave the way for better clinical trials, potentially revolutionizing breast cancer diagnostics worldwide.
You May Also Like: Other Popular Use Cases of AI in Healthcare
How AI in Medicine Improves Patient Care Quality
The precision and customization AI technologies offer lead to more effective therapies, reduced side effects, and improved patient outcomes. Here’s why.
Personalized Disease Treatment
Each patient is different, and AI-powered personalized disease treatment fully embraces that.
AI systems collect data from various sources, including genetic information, electronic health records (EHRs), lifestyle habits, and environmental factors. Large Language Models and Machine learning algorithms analyzed the data to identify patterns and correlations that might not be evident through traditional analysis.

AI tools can analyze genetic data to identify mutations or markers associated with specific diseases. This information allows medical professionals to plan tailored treatment plans that target the genetic basis of a disease.
The treatment also fits the patient’s unique health profile, as established above. By leveraging historical data, AI can predict how a patient might respond to certain treatments. This predictive analysis allows healthcare providers to select therapies with the highest likelihood of success, minimizing trial and error and reducing the risk of adverse reactions.
Better Clinical Trial Efficiency
AI is a support tool in medical practices, providing clinicians with comprehensive, evidence-based insights that enhance decision-making. It streamlines participant recruitment by analyzing large datasets to identify suitable candidates quickly. Its capability to predict patient responses based on genetic and lifestyle information ensures a higher success rate.
Artificial intelligence systems excel at data management and process complex information faster and more accurately. Additionally, AI optimizes trial design through simulations, identifying the best methodologies and reducing the need for costly amendments.
AI-enabled real-time monitoring allows researchers to detect and address issues promptly. These advancements lead to significant cost reductions for trials. Faster and efficient trials result in quicker drug development and availability, allowing patients to access new treatments sooner, especially for urgent medical needs.
Reduced Cost of Care
AI technologies use machine learning algorithms to analyze medical images such as X-rays and MRIs with incredible precision. This reduces diagnostic errors, alleviating the need for additional testing and procedures, which often cost an arm and a leg. AI also delivers results in a fraction of the time it would take through traditional methods. There will be no delayed diagnosis and no additional expenses.
Hospital emergencies and admissions are expensive. With AI-powered predictive analytics, medical professionals and patients alike can take the preemptive strike. Potential health risks and patient deterioration are identified before they become critical, facilitating early intervention.
AI-powered remote monitoring devices and telehealth platforms are redefining patient care. These tools allow continuous monitoring of patient's health from the comfort of their homes. By cutting down on physical visits, AI reduces transportation costs and the load on healthcare facilities, translating to significant savings.
Fewer Human Errors
People make mistakes. That's the human nature. Human errors can stem from various factors, including fatigue, cognitive overload, miscommunication, and complex processes. AI helps mitigate these errors, especially in medical imaging and diagnosis.
AI algorithms give highly accurate readings of X-rays, MRIs, and CT scans by flagging areas of concern that the human eye might overlook. They even exceed human performance in certain diagnostic tasks.
The streamlined access to patient records, treatment plans, and medication prescriptions provides healthcare professionals with actionable insights. Real-time analysis and recommendations induce informed decisions, reducing errors arising from outdated practices or incomplete information.
Accelerated Drug Development
Another area of healthcare that greatly benefits from artificial intelligence is drug discovery and development. The faster the medicine is available on the market, the more patients are saved. The introduction of AI in medicine development has the potential to expedite medication production much more efficiently.
AI algorithms can sift through vast libraries of chemical compounds to identify potential drug candidates. Machine learning models predict which compounds are most likely to interact with specific biological targets, drastically narrowing down the pool of possibilities. This reduces the time and resources required for initial screening.
AI-driven simulations showcase how different molecules interact within the human body. This ensures drug efficacy and safety profiles early in the development process, reducing the need for extensive wet lab experiments.
In clinical trials, AI can enhance the design and management of clinical trials by identifying suitable patient populations, optimizing dosing strategies, and predicting trial outcomes. Machine learning models analyze historical data to forecast which trials are likely to succeed, thus prioritizing resources effectively and shortening the timeline for drug development.
Risks of Using AI in Medicine and Healthcare
The introduction of artificial intelligence in medicine is a double-edged sword. While AI in medicine brings remarkable opportunities for advancement, it also comes with challenges that require thoughtful consideration and proactive management. Here are some of the most prominent risks associated with AI in healthcare.
Data Privacy and Security
AI systems in healthcare handle extremely sensitive information. This includes personal details, medical histories, and treatment plans. If leaked, this data can lead to identity theft and other severe privacy violations. On top of that, any data breach can severely damage the trust patients place in healthcare providers.

Insider threats remain a persistent risk. Employees with access to patients’ sensitive information might misuse it, either intentionally or by accident. This risk highlights the importance of stringent access control measures.
AI systems in healthcare often need to interface with various other IT systems. Each integration point is a potential vulnerability. Attackers can exploit insecure interfaces to gain unauthorized access to sensitive information.
Biased Outcomes
AI models thrive on data. However, if the data used to train these models is biased or incomplete, the outcomes will reflect, if not magnify, those biases. For example, if a dataset predominantly represents one demographic, the AI may perform poorly for others, leading to disparities in healthcare outcomes.
Sometimes, biases emerge not just from the data but from the algorithms themselves. The way algorithms process and prioritize information can introduce unexpected biases. Without careful design and testing, AI systems may deliver wrong positives that could lead to inappropriate treatments, affecting patient care quality.
Lack of Transparency
Many AI systems, particularly those utilizing complex algorithms like deep learning, function as "black boxes." This means that even developers and data scientists often cannot fully understand or explain how the AI arrives at specific conclusions or decisions. In medical settings, this opacity is problematic.
Such a lack of transparency can lead to significant trust issues. Patients and healthcare providers may be wary of relying on AI systems if they do not understand how decisions are made. Hence, stakeholders cannot pinpoint where biases occur and how they influence outcomes.
Informed consent is a cornerstone of ethical medical practice. With AI systems lacking transparency, patients may find it hard to trust a machine’s diagnosis, preventing doctors from assigning timely care.
Regulatory and Ethical Challenges
The healthcare industry operates under strict regulatory frameworks. Laws such as HIPAA in the United States dictate how patient data can be collected, stored, and shared. AI systems must comply with these regulations through interdisciplinary collaboration between technical and legal experts.
Then there is determining accountability. If an AI-driven decision results in an adverse outcome, who would ascertain responsibility? Is it the developers, the healthcare providers, or the institution? Clear guidelines and frameworks are needed to assign responsibility and manage liability in AI-related incidents.
Informed consent, patient autonomy, and potential biases in AI algorithms are other ethical concerns that we have addressed above.
Implementation Costs
AI systems require specific knowledge to operate. Unfortunately, many healthcare providers are not adequately trained to use these technologies. Implementing AI in medicine comes with substantial investment into staff training, alongside already expensive hardware and software.
AI systems must integrate with existing healthcare infrastructure, which also costs big bucks. Add the expenses for maintenance and operation, the bills are prohibitive, especially for smaller practices.
The Future of Artificial Intelligence in Medicine
The world’s healthcare system is on an upward trajectory. People are healthier and live longer today than in the last 30 years. We expect our healthcare will continue to evolve for the better in the foreseeable future, with digital transformation and interoperable data leading the charge. So, what does that mean for artificial intelligence?
Say we venture 30 years into the future. That’s another 30 years' worth of data for machine learning algorithms to analyze. Robots won't replace humans, however cool it might be. Instead, we will combine the services of robots with humans to create more value than we could alone.
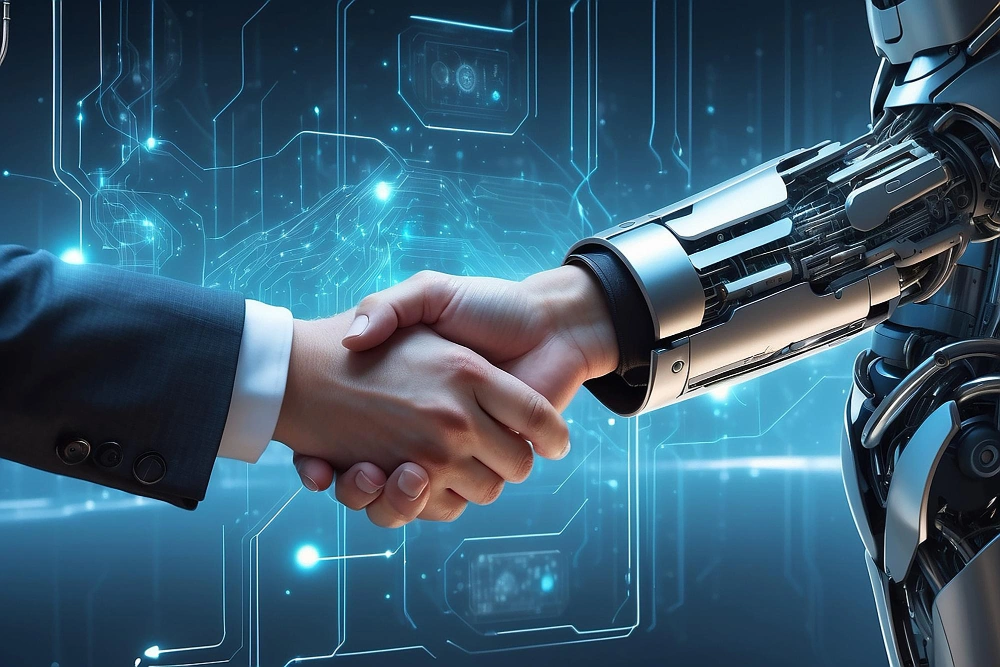
Machine learning helps systems learn better by processing vast amounts of data, which humans can't do alone. Humans, on the other hand, can check the tech's results and focus more on problem-solving tasks.
AI can help reconfigure workspaces and ease burdens on doctors, reducing burnout through tools like drones delivering medical supplies and autonomous vehicles like ambulances improving service efficiency.
AI's use in healthcare is gaining attention, especially in the U.S. and China, but challenges like regulation and cost remain. Innovations, lifestyle changes, and chronic diseases are pushing medicine toward being predictive, preventive, participatory, and personalized.
Stay ahead with Ominext!
The healthcare industry is evolving, and you should too! Staying at the forefront of technology is key to delivering superior patient care and enhancing operational efficiency. At Ominext, we bring over 12 years of specialized experience in medical digital transformation (DX), providing exceptional software development services tailored to the healthcare industry.
Our decade-long journey in the field of medical DX has equipped us with deep expertise and a proven track record of developing innovative solutions that revolutionize healthcare systems. We are well-versed in the complexities of healthcare software, ensuring compliance with industry regulations and seamless integration with existing medical technologies.
Partnering with Ominext means collaborating with a team that combines deep domain knowledge with a commitment to excellence. We leverage cutting-edge technologies to deliver robust, scalable, and secure software solutions that empower healthcare providers to enhance diagnostics, streamline administrative functions, and, most importantly, improve patient outcomes.
Why settle for the ordinary when you can tap into the expertise of a pioneer in medical digital transformation? With Ominext, get ahead in healthcare innovation and let us help you convert your vision into reality—efficiently, reliably, and with the precision that only a decade of focused experience can offer.
Don’t just keep pace with the future of healthcare—lead it. Choose Ominext and discover the transformative difference that true expertise makes.